Cutting Defects, Boosting Yield: The Power of ML in Chip Testing
Discover how ProteanTecs’ machine learning-driven outlier detection is transforming semiconductor testing by improving reliability, reducing waste, and boosting yield.
3/28/20254 min read
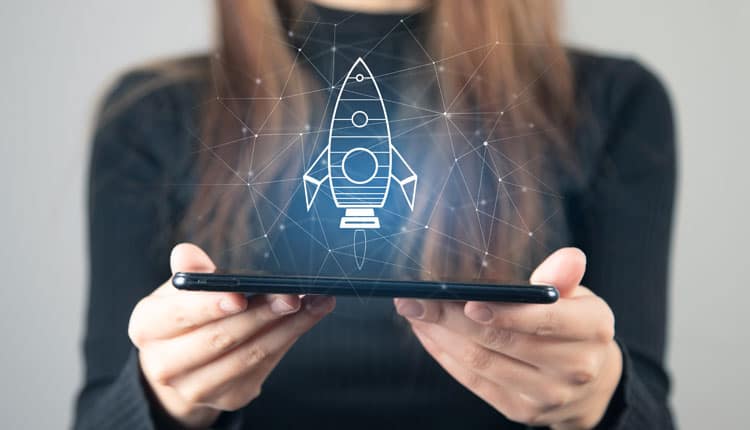
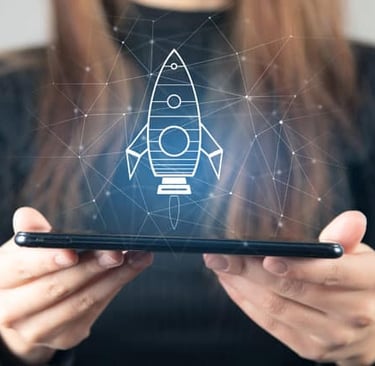
Cut Defects, Not Yield: How Machine Learning is Revolutionizing Semiconductor Testing
In the fast-paced world of semiconductor manufacturing, chipmakers face an ongoing battle: how to ensure the highest levels of quality while maintaining an acceptable yield. It’s a delicate dance between discarding imperfect chips to maintain reliability and risking late-stage failures by letting marginal chips pass through the cracks. What if there was a way to have the best of both worlds? A solution that allowed for rigorous testing and precise detection of latent defects without sacrificing yield?
Enter ProteanTecs — a company that’s shifting the paradigm in outlier detection, using the power of machine learning (ML) and telemetry data to change how chips are tested and ensure reliability without throwing away perfectly good silicon.
The Quest for Reliability: Why Traditional Methods Fall Short
Let’s set the stage. Imagine you’re a chip manufacturer, working at full capacity, cranking out thousands of chips every day. Your goal? To ensure that each one functions flawlessly. To do this, you subject the chips to rigorous tests to catch any possible defects. But here’s the catch — in the process, you’re discarding chips that may be perfectly functional, just slightly outside the strict testing thresholds.
Traditional testing methods like Part Average Testing (PAT) have been the industry standard for years. These methods rely on statistical analysis to improve quality, but they come with inherent limitations. They don’t detect subtle defects. They fail to catch anomalies early, and worse, they may discard healthy chips, exacerbating the delicate balance between quality and yield.
For industries like automotive or data centers, where reliability is critical, the stakes couldn’t be higher. You don’t want to risk letting a defective chip make it to the field — potentially causing system failures, costly return merchandise authorizations (RMAs), or even Silent Data Corruption (SDC), a hidden threat that could take down entire systems without anyone noticing.
So, how do you make sure you catch those elusive defects early without over-testing and reducing yield? That’s where ProteanTecs steps in.
A New Era of Testing: Outlier Detection with ML Precision
What if the future of semiconductor testing wasn’t about tightening thresholds or relying on blunt statistical methods? What if you could rely on machine learning to detect subtle anomalies before they became problems?
ProteanTecs is changing the game with its outlier detection solution that integrates advanced telemetry-based data analytics and machine learning to catch defects early — even at the wafer sort stage.
Here’s how it works:
Telemetry-based Analytics: Embedded agents in the chips continuously collect parametric data during manufacturing. This data is then analyzed by ProteanTecs’ algorithms, which predict the “normal” behavior of a chip.
Machine Learning Models: These models are trained to identify anomalies that traditional tests can’t pick up. By predicting how a chip should behave, the system can flag outliers — those chips that might be just slightly off but still functional — for further review or discarding.
This isn’t just about pass/fail testing anymore; it’s about using data and intelligence to see beyond the obvious and catch defects in the gray areas where traditional tests fail.
Cutting-edge Methods: IDDQ and Timing Margin Detection
ProteanTecs isn’t just relying on basic predictive models; they’ve integrated advanced techniques to further enhance the detection process:
IDDQ Prediction-Based Detection: This method targets leakage current anomalies at the transistor level, detecting issues that would fly under the radar of traditional tests. By combining design profiling and process classification data, ProteanTecs can predict what the expected current should be — and flag chips that don’t meet the mark.
Timing Margin-Based Detection: This method zooms in on timing issues at the transistor and path levels, detecting problems that could lead to timing faults or silent data corruption (SDC). Traditional tests may not catch these issues, but ProteanTecs’ ML models can spot subtle deviations in timing behavior, ensuring that chips with potential problems are identified before they leave the factory.
Why ProteanTecs is a Game Changer
So, why is ProteanTecs’ solution different from traditional testing methods? The secret lies in its ability to go beyond the binary pass/fail logic and dig deep into the data to detect latent defects that would otherwise be missed. The combination of on-chip telemetry agents and predictive machine learning means that chips are tested with unmatched granularity.
Traditional methods simply can’t compete. They rely on strict thresholds and statistical analysis, which may not account for the subtle nuances that can lead to field failures. ProteanTecs, on the other hand, uses real-time data and adaptive algorithms to detect anomalies that might not trigger a traditional fail signal but could still lead to costly failures down the line.
The Benefits: Cutting Waste, Boosting Yield
The benefits of this approach are clear:
Improved Yield: By detecting latent defects early, ProteanTecs ensures that only truly defective chips are discarded, reducing unnecessary waste and boosting yield.
Reduced Testing Costs: By isolating defective chips early, manufacturers can avoid expensive downstream testing, rework, and packaging costs.
Increased Reliability: Early detection of potential defects means fewer late-stage failures, reducing costly RMAs and preventing silent data corruption.
Real-Time Insights: The cloud-based platform provides real-time analytics, enabling proactive decision-making and continuous improvement in the testing process.
Real-World Impact: Success Stories
The real-world results speak for themselves:
Automotive Industry: One chipmaker in the automotive sector reduced Defective Parts Per Million (DPPM) by an impressive 396, saving over $250,000 in testing and packaging costs. The effectiveness was validated through High-Temperature Operating Life (HTOL) tests, with 70% of flagged outliers failing stress tests.
Data Centers: A networking chipmaker serving data centers cut DPPM by 252, avoiding latent defects that could have led to costly system failures. This early detection saved over $1 million, ensuring the uninterrupted performance of critical systems.
The Future of Semiconductor Testing
ProteanTecs represents a new era in semiconductor testing — one where precision, adaptability, and efficiency come together to transform the way we approach quality and yield in chip manufacturing.
For chipmakers operating in industries where reliability and performance are non-negotiable, ProteanTecs’ outlier detection solution is more than just a luxury; it’s a necessity. By harnessing the power of machine learning and real-time data, manufacturers can now ensure the highest levels of quality while minimizing waste and avoiding costly failures down the line.
In a world where defects and yield are constantly at odds, ProteanTecs is helping chipmakers cut defects without cutting yield. And that, in the world of semiconductor manufacturing, is nothing short of revolutionary.
Source - Semiwiki
QUICK LINKS
NEIL RAO TOWERS, 117 & 118, Rd Number 3, Vijayanagar, EPIP Zone, Whitefield, Bengaluru, Karnataka 560066
200/2, Tada Kandriga, Tada Mandalam, Tirupati District - 524401
Locations